This pipeline computes the correlation between significant copy number variation (cnv) genes and selected clinical features.
Testing the association between copy number variation of 17 peak regions and 8 clinical features across 95 patients, 2 significant findings detected with Q value < 0.25.
-
Del Peak 16(Xq21.31) cnvs correlated to 'Time to Death'.
-
Del Peak 17(Xq28) cnvs correlated to 'Time to Death'.
Table 1. Get Full Table Overview of the association between significant copy number variation of 17 regions and 8 clinical features. Shown in the table are P values (Q values). Thresholded by Q value < 0.25, 2 significant findings detected.
Clinical Features |
Time to Death |
AGE | GENDER |
KARNOFSKY PERFORMANCE SCORE |
PATHOLOGY T |
PATHOLOGY N |
PATHOLOGICSPREAD(M) |
TUMOR STAGE |
||
nCNV (%) | nWild-Type | logrank test | t-test | Fisher's exact test | t-test | Fisher's exact test | Fisher's exact test | Fisher's exact test | Fisher's exact test | |
Del Peak 16(Xq21 31) | 12 (13%) | 83 |
3.65e-05 (0.00457) |
0.148 (1.00) |
0.328 (1.00) |
0.466 (1.00) |
0.0321 (1.00) |
0.714 (1.00) |
0.223 (1.00) |
0.121 (1.00) |
Del Peak 17(Xq28) | 11 (12%) | 84 |
7.66e-06 (0.000965) |
0.204 (1.00) |
0.291 (1.00) |
0.498 (1.00) |
0.241 (1.00) |
0.49 (1.00) |
0.726 (1.00) |
0.341 (1.00) |
Amp Peak 1(19p13 2) | 11 (12%) | 84 |
0.99 (1.00) |
0.176 (1.00) |
1 (1.00) |
0.152 (1.00) |
0.0558 (1.00) |
0.687 (1.00) |
0.826 (1.00) |
0.579 (1.00) |
Del Peak 1(1p36 31) | 18 (19%) | 77 |
0.206 (1.00) |
0.415 (1.00) |
0.776 (1.00) |
0.422 (1.00) |
0.0802 (1.00) |
0.406 (1.00) |
0.0078 (0.959) |
0.0507 (1.00) |
Del Peak 2(2p11 2) | 3 (3%) | 92 |
0.355 (1.00) |
0.686 (1.00) |
1 (1.00) |
0.68 (1.00) |
1 (1.00) |
0.552 (1.00) |
||
Del Peak 3(2q37 3) | 5 (5%) | 90 |
0.656 (1.00) |
0.357 (1.00) |
0.15 (1.00) |
0.0141 (1.00) |
0.448 (1.00) |
0.0175 (1.00) |
||
Del Peak 4(3p22 1) | 5 (5%) | 90 |
0.216 (1.00) |
0.106 (1.00) |
0.317 (1.00) |
0.164 (1.00) |
1 (1.00) |
0.385 (1.00) |
0.105 (1.00) |
|
Del Peak 5(4q32 1) | 10 (11%) | 85 |
0.0681 (1.00) |
0.605 (1.00) |
1 (1.00) |
0.429 (1.00) |
0.763 (1.00) |
0.152 (1.00) |
0.359 (1.00) |
|
Del Peak 6(5p15 33) | 7 (7%) | 88 |
0.111 (1.00) |
0.339 (1.00) |
0.417 (1.00) |
0.036 (1.00) |
0.0263 (1.00) |
0.428 (1.00) |
0.23 (1.00) |
|
Del Peak 7(5q15) | 7 (7%) | 88 |
0.615 (1.00) |
0.123 (1.00) |
0.417 (1.00) |
0.0953 (1.00) |
0.509 (1.00) |
0.136 (1.00) |
0.361 (1.00) |
|
Del Peak 8(5q35 2) | 7 (7%) | 88 |
0.615 (1.00) |
0.233 (1.00) |
0.417 (1.00) |
0.036 (1.00) |
0.509 (1.00) |
0.428 (1.00) |
0.0867 (1.00) |
|
Del Peak 9(6q22 31) | 10 (11%) | 85 |
0.0284 (1.00) |
0.73 (1.00) |
0.0595 (1.00) |
0.356 (1.00) |
0.0449 (1.00) |
0.816 (1.00) |
0.204 (1.00) |
0.223 (1.00) |
Del Peak 10(9p21 3) | 15 (16%) | 80 |
0.0059 (0.732) |
0.545 (1.00) |
0.0352 (1.00) |
0.422 (1.00) |
0.0112 (1.00) |
0.729 (1.00) |
0.0322 (1.00) |
0.0271 (1.00) |
Del Peak 12(14q11 2) | 20 (21%) | 75 |
0.223 (1.00) |
0.423 (1.00) |
0.586 (1.00) |
0.473 (1.00) |
0.157 (1.00) |
0.656 (1.00) |
0.0948 (1.00) |
0.0789 (1.00) |
Del Peak 13(14q23 3) | 19 (20%) | 76 |
0.203 (1.00) |
0.821 (1.00) |
0.574 (1.00) |
0.422 (1.00) |
0.0923 (1.00) |
0.243 (1.00) |
0.107 (1.00) |
0.0474 (1.00) |
Del Peak 14(14q32 2) | 19 (20%) | 76 |
0.203 (1.00) |
0.776 (1.00) |
0.786 (1.00) |
0.422 (1.00) |
0.311 (1.00) |
0.243 (1.00) |
0.0561 (1.00) |
0.0551 (1.00) |
Del Peak 15(19q13 42) | 5 (5%) | 90 |
0.422 (1.00) |
0.333 (1.00) |
1 (1.00) |
0.0877 (1.00) |
0.687 (1.00) |
0.448 (1.00) |
0.204 (1.00) |
P value = 3.65e-05 (logrank test), Q value = 0.0046
Table S1. Gene #16: 'Del Peak 16(Xq21.31) mutation analysis' versus Clinical Feature #1: 'Time to Death'
nPatients | nDeath | Duration Range (Median), Month | |
---|---|---|---|
ALL | 88 | 14 | 0.0 - 182.7 (15.7) |
DEL PEAK 16(XQ21.31) MUTATED | 11 | 5 | 0.0 - 79.8 (9.6) |
DEL PEAK 16(XQ21.31) WILD-TYPE | 77 | 9 | 0.5 - 182.7 (20.1) |
Figure S1. Get High-res Image Gene #16: 'Del Peak 16(Xq21.31) mutation analysis' versus Clinical Feature #1: 'Time to Death'
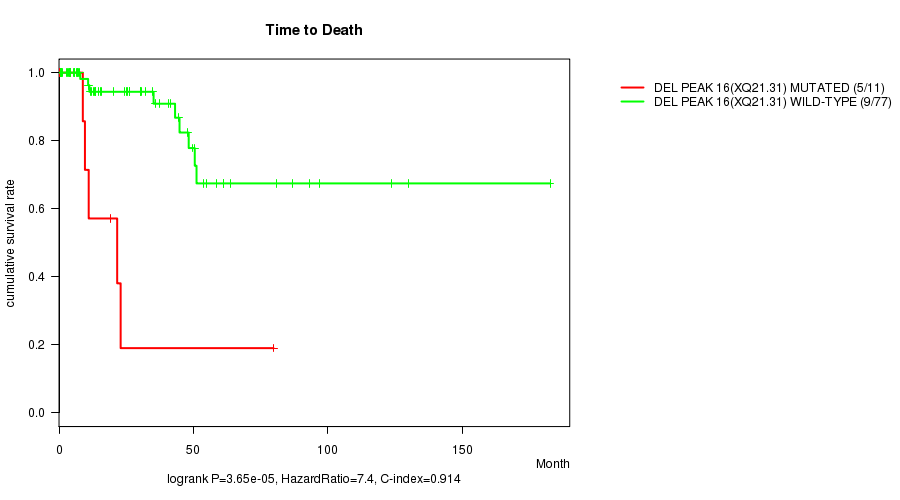
P value = 7.66e-06 (logrank test), Q value = 0.00097
Table S2. Gene #17: 'Del Peak 17(Xq28) mutation analysis' versus Clinical Feature #1: 'Time to Death'
nPatients | nDeath | Duration Range (Median), Month | |
---|---|---|---|
ALL | 88 | 14 | 0.0 - 182.7 (15.7) |
DEL PEAK 17(XQ28) MUTATED | 10 | 4 | 0.0 - 22.9 (10.3) |
DEL PEAK 17(XQ28) WILD-TYPE | 78 | 10 | 0.5 - 182.7 (22.3) |
Figure S2. Get High-res Image Gene #17: 'Del Peak 17(Xq28) mutation analysis' versus Clinical Feature #1: 'Time to Death'
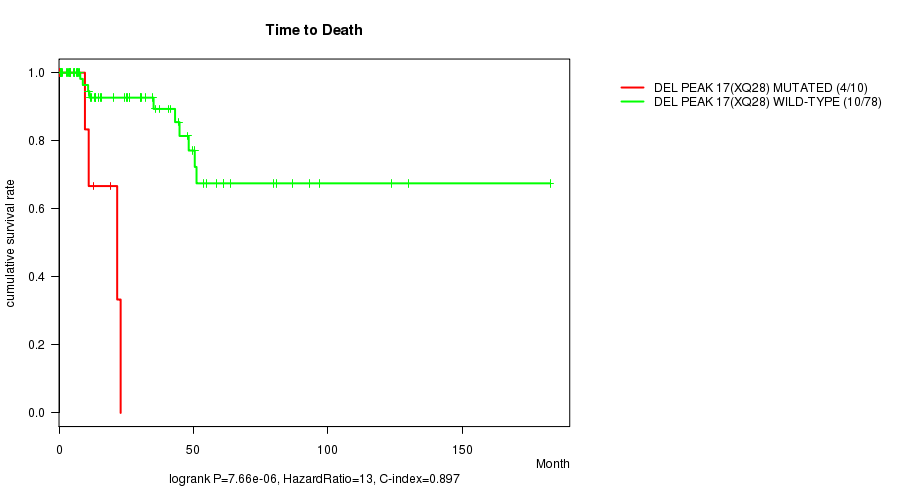
-
Copy number data file = All Lesions File (all_lesions.conf_##.txt, where ## is the confidence level). The all lesions file is from GISTIC pipeline and summarizes the results from the GISTIC run. It contains data about the significant regions of amplification and deletion as well as which samples are amplified or deleted in each of these regions. The identified regions are listed down the first column, and the samples are listed across the first row, starting in column 10.
-
Clinical data file = KIRP.clin.merged.picked.txt
-
Number of patients = 95
-
Number of copy number variation regions = 17
-
Number of selected clinical features = 8
-
Exclude regions that fewer than K tumors have alterations, K = 3
For survival clinical features, the Kaplan-Meier survival curves of tumors with and without gene cnvs were plotted and the statistical significance P values were estimated by logrank test (Bland and Altman 2004) using the 'survdiff' function in R
For continuous numerical clinical features, two-tailed Student's t test with unequal variance (Lehmann and Romano 2005) was applied to compare the clinical values between tumors with and without gene cnvs using 't.test' function in R
For binary or multi-class clinical features (nominal or ordinal), two-tailed Fisher's exact tests (Fisher 1922) were used to estimate the P values using the 'fisher.test' function in R
For multiple hypothesis correction, Q value is the False Discovery Rate (FDR) analogue of the P value (Benjamini and Hochberg 1995), defined as the minimum FDR at which the test may be called significant. We used the 'Benjamini and Hochberg' method of 'p.adjust' function in R to convert P values into Q values.
This is an experimental feature. The full results of the analysis summarized in this report can be downloaded from the TCGA Data Coordination Center.