This pipeline computes the correlation between cancer subtypes identified by different molecular patterns and selected clinical features.
Testing the association between subtypes identified by 6 different clustering approaches and 4 clinical features across 124 patients, 2 significant findings detected with P value < 0.05.
-
3 subtypes identified in current cancer cohort by 'CN CNMF'. These subtypes do not correlate to any clinical features.
-
3 subtypes identified in current cancer cohort by 'METHLYATION CNMF'. These subtypes correlate to 'AGE'.
-
CNMF clustering analysis on sequencing-based mRNA expression data identified 9 subtypes that do not correlate to any clinical features.
-
Consensus hierarchical clustering analysis on sequencing-based mRNA expression data identified 4 subtypes that correlate to 'NEOADJUVANT.THERAPY'.
-
CNMF clustering analysis on sequencing-based miR expression data identified 4 subtypes that do not correlate to any clinical features.
-
Consensus hierarchical clustering analysis on sequencing-based miR expression data identified 3 subtypes that do not correlate to any clinical features.
Table 1. Get Full Table Overview of the association between subtypes identified by 6 different clustering approaches and 4 clinical features. Shown in the table are P values from statistical tests. Thresholded by P value < 0.05, 2 significant findings detected.
Clinical Features |
Time to Death |
AGE |
RADIATIONS RADIATION REGIMENINDICATION |
NEOADJUVANT THERAPY |
Statistical Tests | logrank test | ANOVA | Fisher's exact test | Fisher's exact test |
CN CNMF | 1 | 0.471 | 1 | 0.666 |
METHLYATION CNMF | 1 | 0.00334 | 1 | 0.315 |
RNAseq CNMF subtypes | 1 | 0.057 | 0.61 | 0.252 |
RNAseq cHierClus subtypes | 1 | 0.0522 | 1 | 0.0124 |
MIRseq CNMF subtypes | 1 | 0.306 | 1 | 0.249 |
MIRseq cHierClus subtypes | 1 | 0.138 | 1 | 0.145 |
Table S1. Get Full Table Description of clustering approach #1: 'CN CNMF'
Cluster Labels | 1 | 2 | 3 |
---|---|---|---|
Number of samples | 24 | 61 | 38 |
P value = 1 (logrank test)
Table S2. Clustering Approach #1: 'CN CNMF' versus Clinical Feature #1: 'Time to Death'
nPatients | nDeath | Duration Range (Median), Month | |
---|---|---|---|
ALL | 123 | 1 | 0.3 - 66.0 (19.5) |
subtype1 | 24 | 0 | 0.3 - 61.1 (12.0) |
subtype2 | 61 | 0 | 1.0 - 65.9 (24.0) |
subtype3 | 38 | 1 | 0.9 - 66.0 (17.9) |
Figure S1. Get High-res Image Clustering Approach #1: 'CN CNMF' versus Clinical Feature #1: 'Time to Death'
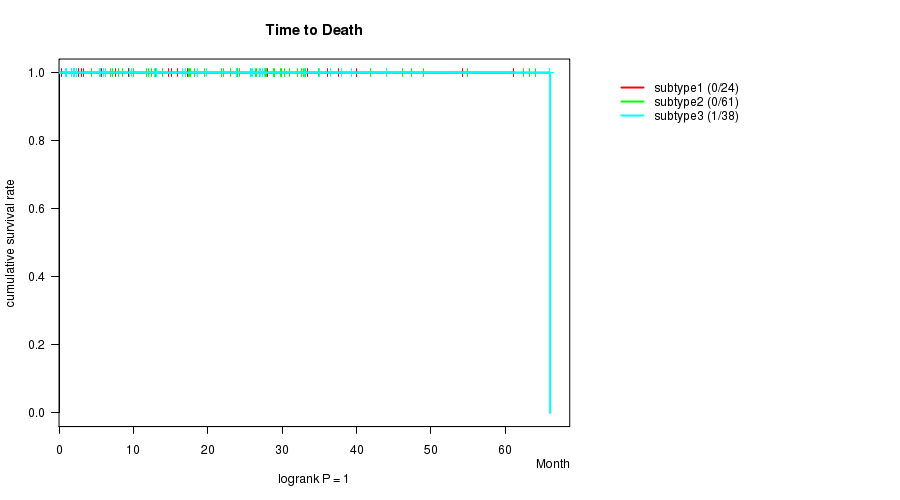
P value = 0.471 (ANOVA)
Table S3. Clustering Approach #1: 'CN CNMF' versus Clinical Feature #2: 'AGE'
nPatients | Mean (Std.Dev) | |
---|---|---|
ALL | 123 | 61.1 (6.6) |
subtype1 | 24 | 61.6 (6.7) |
subtype2 | 61 | 60.4 (7.0) |
subtype3 | 38 | 62.0 (6.0) |
Figure S2. Get High-res Image Clustering Approach #1: 'CN CNMF' versus Clinical Feature #2: 'AGE'
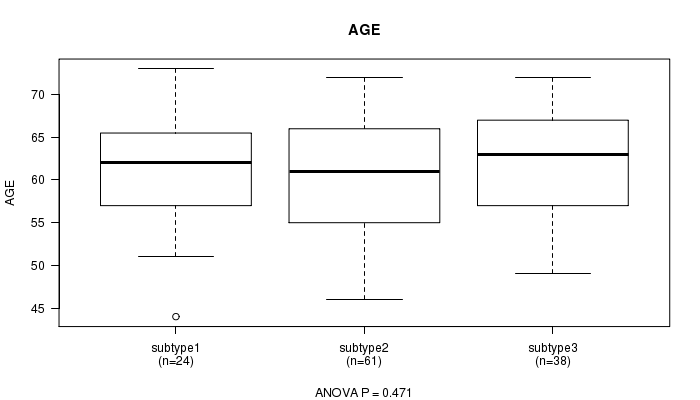
P value = 1 (Fisher's exact test)
Table S4. Clustering Approach #1: 'CN CNMF' versus Clinical Feature #3: 'RADIATIONS.RADIATION.REGIMENINDICATION'
nPatients | NO | YES |
---|---|---|
ALL | 5 | 118 |
subtype1 | 1 | 23 |
subtype2 | 3 | 58 |
subtype3 | 1 | 37 |
Figure S3. Get High-res Image Clustering Approach #1: 'CN CNMF' versus Clinical Feature #3: 'RADIATIONS.RADIATION.REGIMENINDICATION'
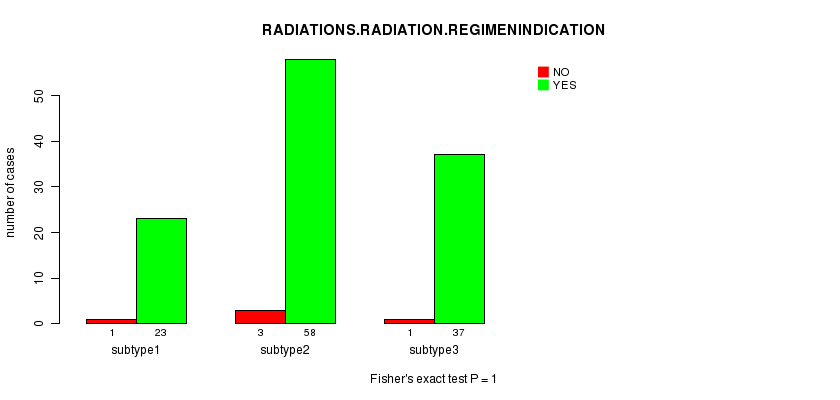
P value = 0.666 (Fisher's exact test)
Table S5. Clustering Approach #1: 'CN CNMF' versus Clinical Feature #4: 'NEOADJUVANT.THERAPY'
nPatients | NO | YES |
---|---|---|
ALL | 4 | 119 |
subtype1 | 0 | 24 |
subtype2 | 2 | 59 |
subtype3 | 2 | 36 |
Figure S4. Get High-res Image Clustering Approach #1: 'CN CNMF' versus Clinical Feature #4: 'NEOADJUVANT.THERAPY'
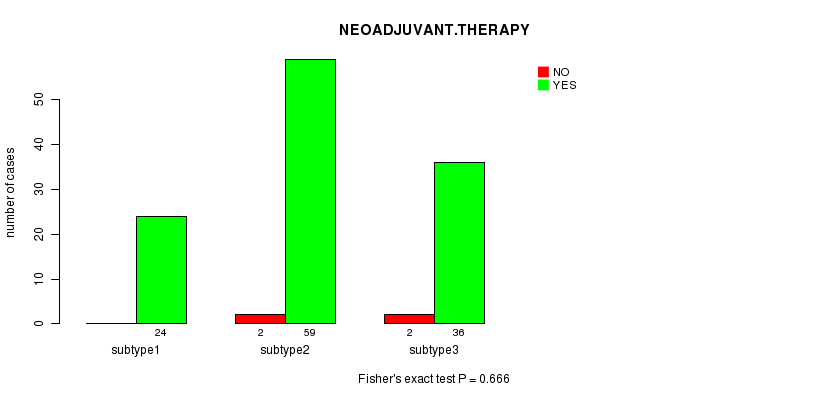
Table S6. Get Full Table Description of clustering approach #2: 'METHLYATION CNMF'
Cluster Labels | 1 | 2 | 3 |
---|---|---|---|
Number of samples | 36 | 41 | 47 |
P value = 1 (logrank test)
Table S7. Clustering Approach #2: 'METHLYATION CNMF' versus Clinical Feature #1: 'Time to Death'
nPatients | nDeath | Duration Range (Median), Month | |
---|---|---|---|
ALL | 124 | 1 | 0.3 - 66.0 (19.1) |
subtype1 | 36 | 0 | 0.3 - 65.9 (21.0) |
subtype2 | 41 | 0 | 1.0 - 62.4 (17.6) |
subtype3 | 47 | 1 | 1.0 - 66.0 (19.5) |
Figure S5. Get High-res Image Clustering Approach #2: 'METHLYATION CNMF' versus Clinical Feature #1: 'Time to Death'
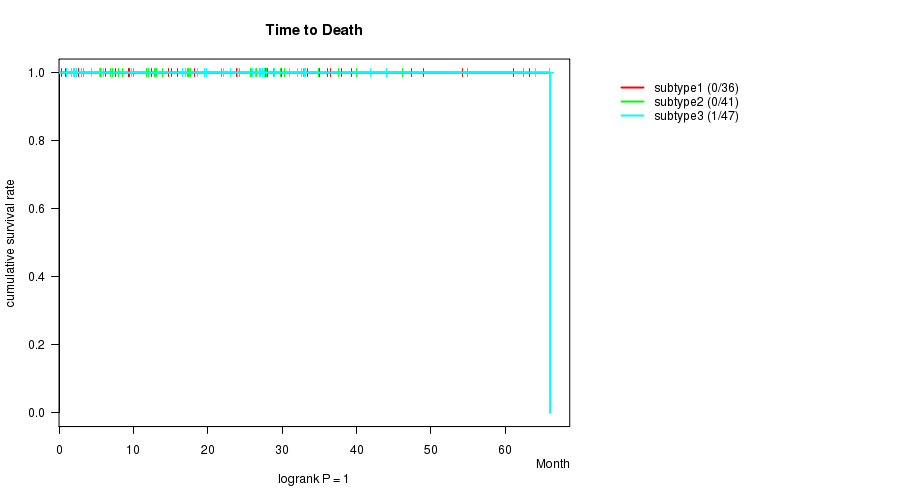
P value = 0.00334 (ANOVA)
Table S8. Clustering Approach #2: 'METHLYATION CNMF' versus Clinical Feature #2: 'AGE'
nPatients | Mean (Std.Dev) | |
---|---|---|
ALL | 124 | 61.2 (6.6) |
subtype1 | 36 | 62.9 (5.7) |
subtype2 | 41 | 58.4 (7.0) |
subtype3 | 47 | 62.3 (6.2) |
Figure S6. Get High-res Image Clustering Approach #2: 'METHLYATION CNMF' versus Clinical Feature #2: 'AGE'
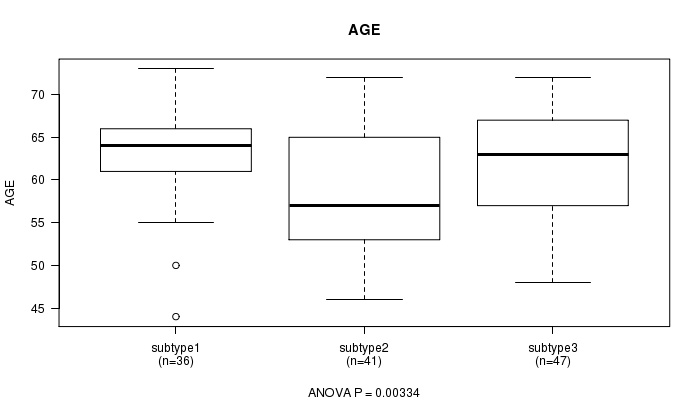
P value = 1 (Fisher's exact test)
Table S9. Clustering Approach #2: 'METHLYATION CNMF' versus Clinical Feature #3: 'RADIATIONS.RADIATION.REGIMENINDICATION'
nPatients | NO | YES |
---|---|---|
ALL | 5 | 119 |
subtype1 | 1 | 35 |
subtype2 | 2 | 39 |
subtype3 | 2 | 45 |
Figure S7. Get High-res Image Clustering Approach #2: 'METHLYATION CNMF' versus Clinical Feature #3: 'RADIATIONS.RADIATION.REGIMENINDICATION'
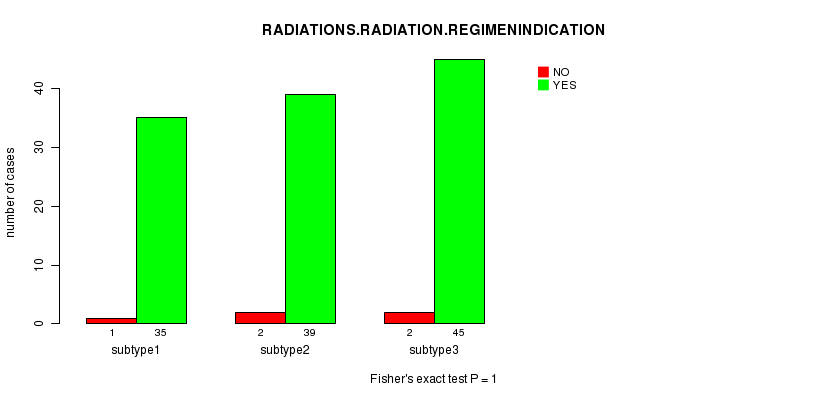
P value = 0.315 (Fisher's exact test)
Table S10. Clustering Approach #2: 'METHLYATION CNMF' versus Clinical Feature #4: 'NEOADJUVANT.THERAPY'
nPatients | NO | YES |
---|---|---|
ALL | 4 | 120 |
subtype1 | 1 | 35 |
subtype2 | 0 | 41 |
subtype3 | 3 | 44 |
Figure S8. Get High-res Image Clustering Approach #2: 'METHLYATION CNMF' versus Clinical Feature #4: 'NEOADJUVANT.THERAPY'
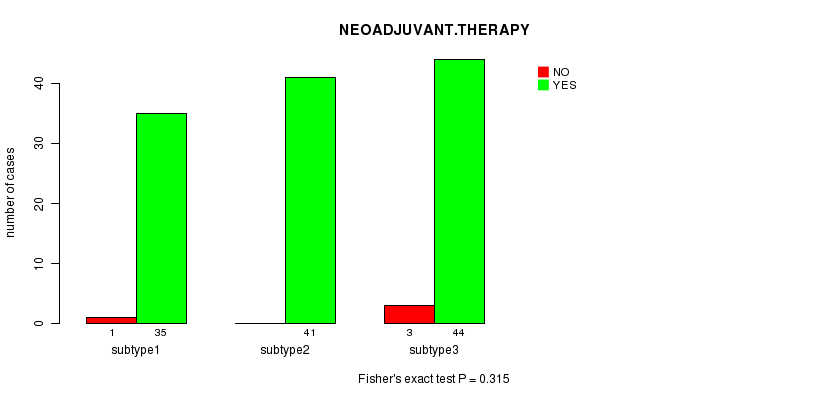
Table S11. Get Full Table Description of clustering approach #3: 'RNAseq CNMF subtypes'
Cluster Labels | 1 | 2 | 3 | 4 | 5 | 6 | 7 | 8 | 9 |
---|---|---|---|---|---|---|---|---|---|
Number of samples | 7 | 3 | 7 | 7 | 8 | 5 | 4 | 8 | 4 |
P value = 1 (logrank test)
Table S12. Clustering Approach #3: 'RNAseq CNMF subtypes' versus Clinical Feature #1: 'Time to Death'
nPatients | nDeath | Duration Range (Median), Month | |
---|---|---|---|
ALL | 53 | 0 | 1.0 - 65.9 (21.8) |
subtype1 | 7 | 0 | 3.0 - 47.4 (15.9) |
subtype2 | 3 | 0 | 1.0 - 44.0 (7.0) |
subtype3 | 7 | 0 | 2.0 - 35.0 (24.0) |
subtype4 | 7 | 0 | 1.0 - 65.9 (19.5) |
subtype5 | 8 | 0 | 1.0 - 34.9 (13.9) |
subtype6 | 5 | 0 | 12.0 - 27.9 (26.4) |
subtype7 | 4 | 0 | 1.0 - 33.0 (11.3) |
subtype8 | 8 | 0 | 13.1 - 46.1 (22.2) |
subtype9 | 4 | 0 | 17.5 - 54.9 (35.2) |
Figure S9. Get High-res Image Clustering Approach #3: 'RNAseq CNMF subtypes' versus Clinical Feature #1: 'Time to Death'
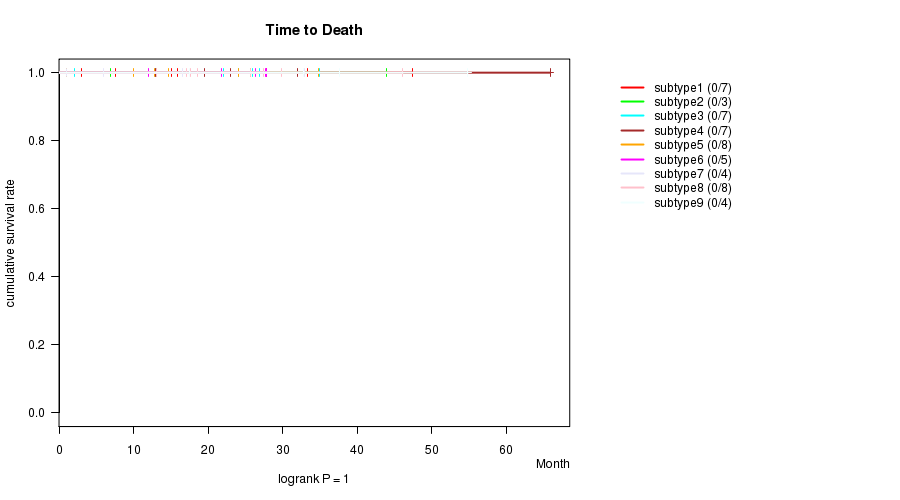
P value = 0.057 (ANOVA)
Table S13. Clustering Approach #3: 'RNAseq CNMF subtypes' versus Clinical Feature #2: 'AGE'
nPatients | Mean (Std.Dev) | |
---|---|---|
ALL | 53 | 61.3 (7.2) |
subtype1 | 7 | 64.6 (4.7) |
subtype2 | 3 | 67.3 (6.4) |
subtype3 | 7 | 65.9 (8.3) |
subtype4 | 7 | 58.9 (4.5) |
subtype5 | 8 | 55.8 (6.3) |
subtype6 | 5 | 63.6 (8.6) |
subtype7 | 4 | 62.2 (9.1) |
subtype8 | 8 | 57.9 (6.7) |
subtype9 | 4 | 61.5 (4.7) |
Figure S10. Get High-res Image Clustering Approach #3: 'RNAseq CNMF subtypes' versus Clinical Feature #2: 'AGE'
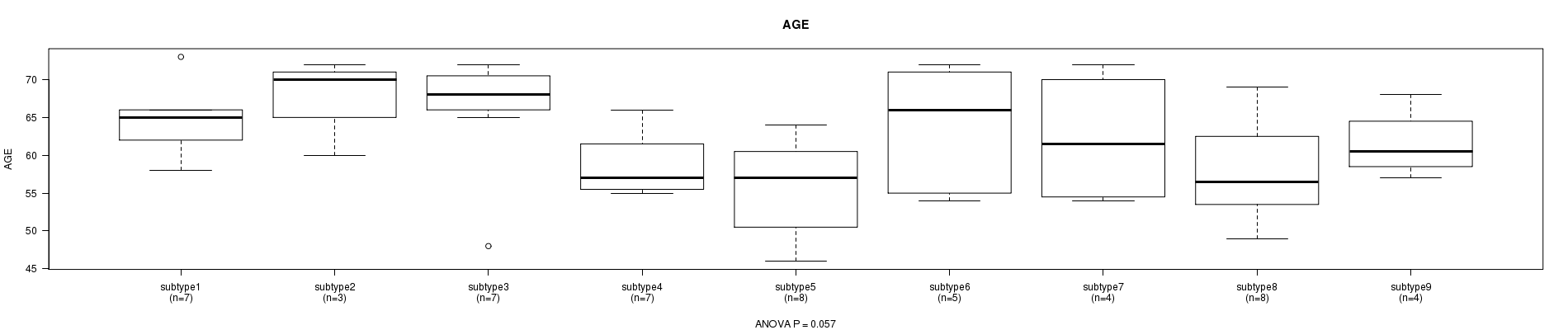
P value = 0.61 (Chi-square test)
Table S14. Clustering Approach #3: 'RNAseq CNMF subtypes' versus Clinical Feature #3: 'RADIATIONS.RADIATION.REGIMENINDICATION'
nPatients | NO | YES |
---|---|---|
ALL | 4 | 49 |
subtype1 | 1 | 6 |
subtype2 | 1 | 2 |
subtype3 | 0 | 7 |
subtype4 | 1 | 6 |
subtype5 | 1 | 7 |
subtype6 | 0 | 5 |
subtype7 | 0 | 4 |
subtype8 | 0 | 8 |
subtype9 | 0 | 4 |
Figure S11. Get High-res Image Clustering Approach #3: 'RNAseq CNMF subtypes' versus Clinical Feature #3: 'RADIATIONS.RADIATION.REGIMENINDICATION'
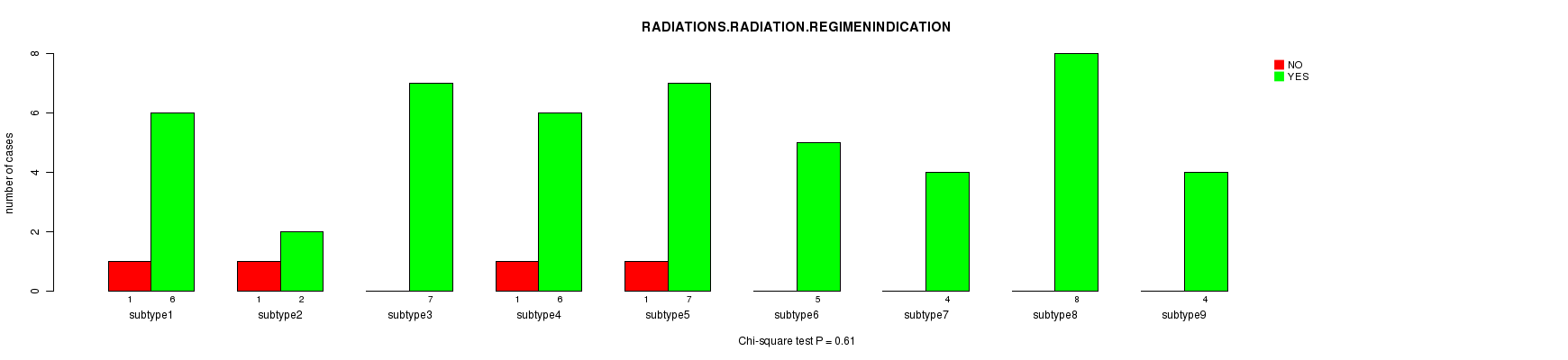
P value = 0.252 (Chi-square test)
Table S15. Clustering Approach #3: 'RNAseq CNMF subtypes' versus Clinical Feature #4: 'NEOADJUVANT.THERAPY'
nPatients | NO | YES |
---|---|---|
ALL | 3 | 50 |
subtype1 | 0 | 7 |
subtype2 | 0 | 3 |
subtype3 | 2 | 5 |
subtype4 | 1 | 6 |
subtype5 | 0 | 8 |
subtype6 | 0 | 5 |
subtype7 | 0 | 4 |
subtype8 | 0 | 8 |
subtype9 | 0 | 4 |
Figure S12. Get High-res Image Clustering Approach #3: 'RNAseq CNMF subtypes' versus Clinical Feature #4: 'NEOADJUVANT.THERAPY'
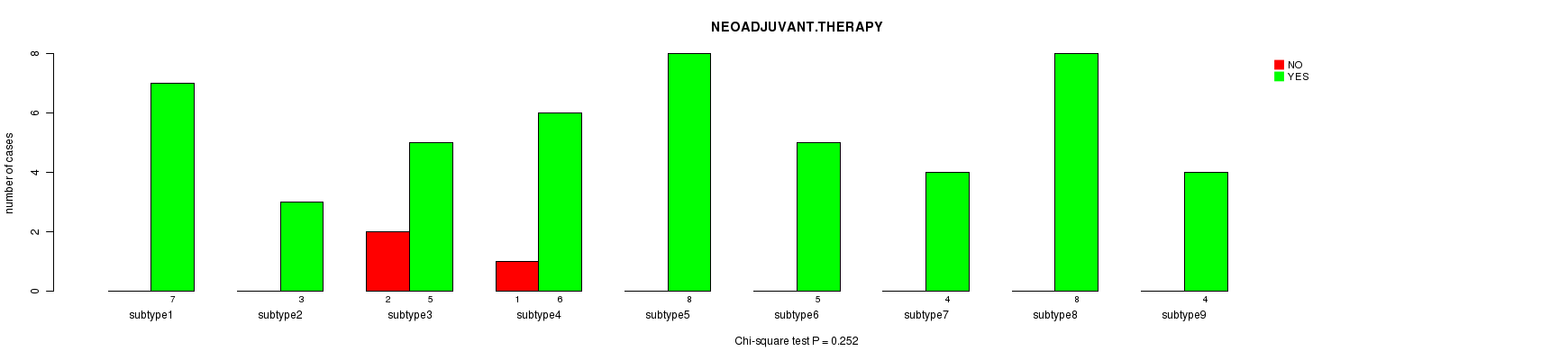
Table S16. Get Full Table Description of clustering approach #4: 'RNAseq cHierClus subtypes'
Cluster Labels | 1 | 2 | 3 | 4 |
---|---|---|---|---|
Number of samples | 11 | 10 | 6 | 26 |
P value = 1 (logrank test)
Table S17. Clustering Approach #4: 'RNAseq cHierClus subtypes' versus Clinical Feature #1: 'Time to Death'
nPatients | nDeath | Duration Range (Median), Month | |
---|---|---|---|
ALL | 53 | 0 | 1.0 - 65.9 (21.8) |
subtype1 | 11 | 0 | 1.0 - 47.4 (15.9) |
subtype2 | 10 | 0 | 1.0 - 65.9 (14.8) |
subtype3 | 6 | 0 | 1.0 - 35.0 (24.0) |
subtype4 | 26 | 0 | 1.0 - 54.9 (23.5) |
Figure S13. Get High-res Image Clustering Approach #4: 'RNAseq cHierClus subtypes' versus Clinical Feature #1: 'Time to Death'
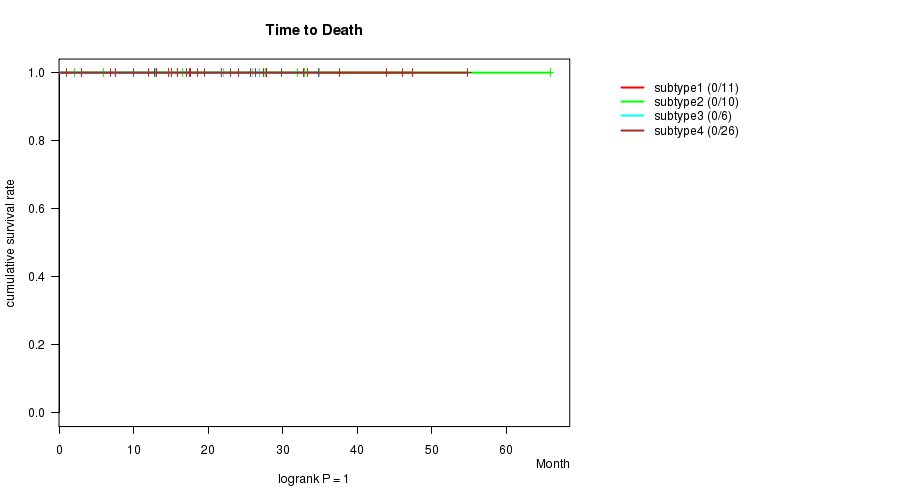
P value = 0.0522 (ANOVA)
Table S18. Clustering Approach #4: 'RNAseq cHierClus subtypes' versus Clinical Feature #2: 'AGE'
nPatients | Mean (Std.Dev) | |
---|---|---|
ALL | 53 | 61.3 (7.2) |
subtype1 | 11 | 62.0 (5.7) |
subtype2 | 10 | 60.1 (8.3) |
subtype3 | 6 | 68.5 (2.4) |
subtype4 | 26 | 59.8 (7.3) |
Figure S14. Get High-res Image Clustering Approach #4: 'RNAseq cHierClus subtypes' versus Clinical Feature #2: 'AGE'
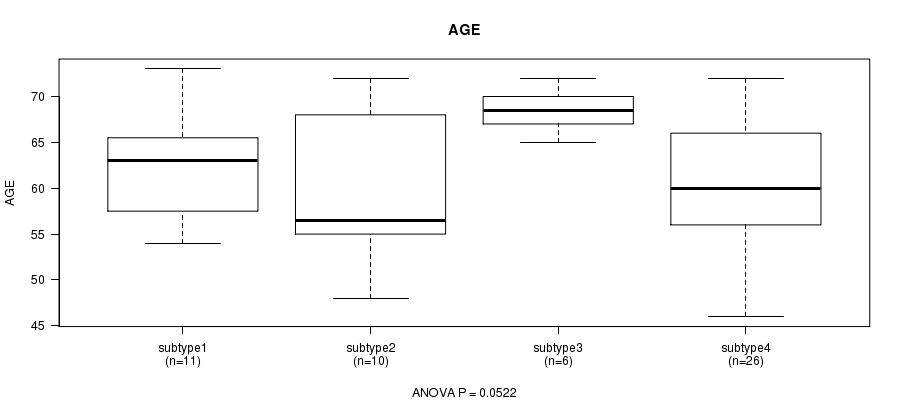
P value = 1 (Fisher's exact test)
Table S19. Clustering Approach #4: 'RNAseq cHierClus subtypes' versus Clinical Feature #3: 'RADIATIONS.RADIATION.REGIMENINDICATION'
nPatients | NO | YES |
---|---|---|
ALL | 4 | 49 |
subtype1 | 1 | 10 |
subtype2 | 1 | 9 |
subtype3 | 0 | 6 |
subtype4 | 2 | 24 |
Figure S15. Get High-res Image Clustering Approach #4: 'RNAseq cHierClus subtypes' versus Clinical Feature #3: 'RADIATIONS.RADIATION.REGIMENINDICATION'
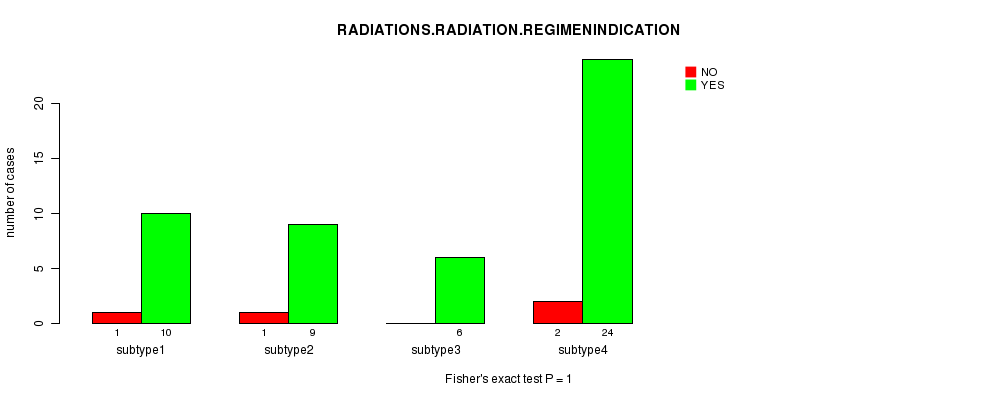
P value = 0.0124 (Fisher's exact test)
Table S20. Clustering Approach #4: 'RNAseq cHierClus subtypes' versus Clinical Feature #4: 'NEOADJUVANT.THERAPY'
nPatients | NO | YES |
---|---|---|
ALL | 3 | 50 |
subtype1 | 0 | 11 |
subtype2 | 1 | 9 |
subtype3 | 2 | 4 |
subtype4 | 0 | 26 |
Figure S16. Get High-res Image Clustering Approach #4: 'RNAseq cHierClus subtypes' versus Clinical Feature #4: 'NEOADJUVANT.THERAPY'
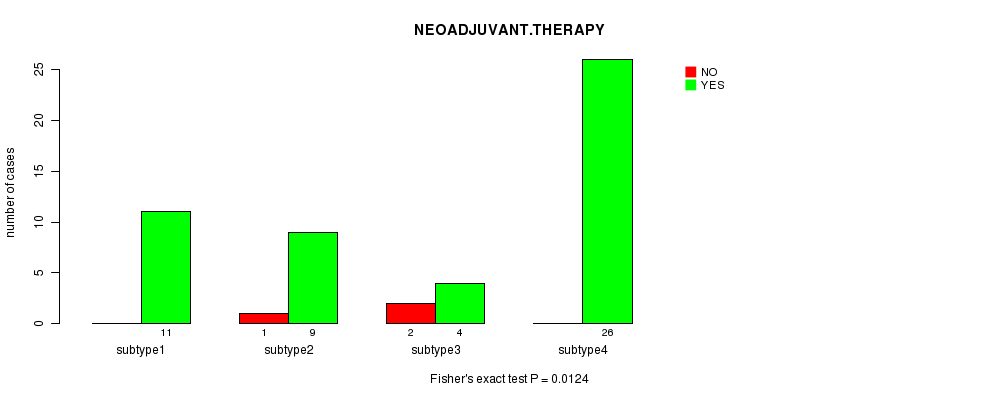
Table S21. Get Full Table Description of clustering approach #5: 'MIRseq CNMF subtypes'
Cluster Labels | 1 | 2 | 3 | 4 |
---|---|---|---|---|
Number of samples | 18 | 28 | 19 | 16 |
P value = 1 (logrank test)
Table S22. Clustering Approach #5: 'MIRseq CNMF subtypes' versus Clinical Feature #1: 'Time to Death'
nPatients | nDeath | Duration Range (Median), Month | |
---|---|---|---|
ALL | 81 | 0 | 0.9 - 65.9 (23.0) |
subtype1 | 18 | 0 | 1.0 - 65.9 (20.8) |
subtype2 | 28 | 0 | 0.9 - 39.3 (21.2) |
subtype3 | 19 | 0 | 11.7 - 54.9 (19.9) |
subtype4 | 16 | 0 | 8.0 - 47.4 (26.2) |
Figure S17. Get High-res Image Clustering Approach #5: 'MIRseq CNMF subtypes' versus Clinical Feature #1: 'Time to Death'
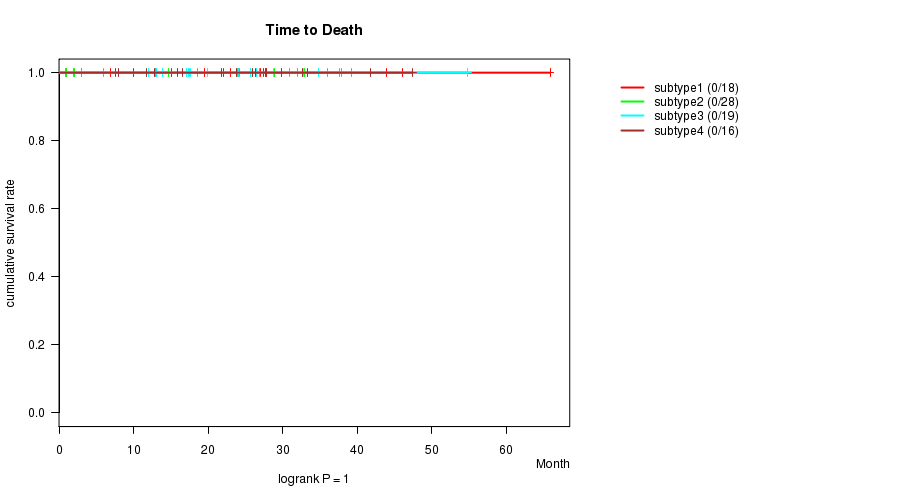
P value = 0.306 (ANOVA)
Table S23. Clustering Approach #5: 'MIRseq CNMF subtypes' versus Clinical Feature #2: 'AGE'
nPatients | Mean (Std.Dev) | |
---|---|---|
ALL | 81 | 61.1 (6.7) |
subtype1 | 18 | 62.7 (6.7) |
subtype2 | 28 | 61.6 (6.3) |
subtype3 | 19 | 58.7 (7.1) |
subtype4 | 16 | 61.4 (6.7) |
Figure S18. Get High-res Image Clustering Approach #5: 'MIRseq CNMF subtypes' versus Clinical Feature #2: 'AGE'
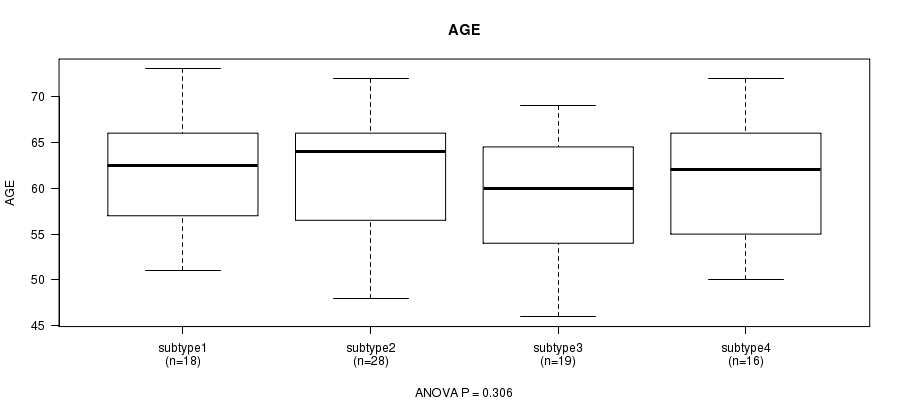
P value = 1 (Fisher's exact test)
Table S24. Clustering Approach #5: 'MIRseq CNMF subtypes' versus Clinical Feature #3: 'RADIATIONS.RADIATION.REGIMENINDICATION'
nPatients | NO | YES |
---|---|---|
ALL | 5 | 76 |
subtype1 | 1 | 17 |
subtype2 | 2 | 26 |
subtype3 | 1 | 18 |
subtype4 | 1 | 15 |
Figure S19. Get High-res Image Clustering Approach #5: 'MIRseq CNMF subtypes' versus Clinical Feature #3: 'RADIATIONS.RADIATION.REGIMENINDICATION'
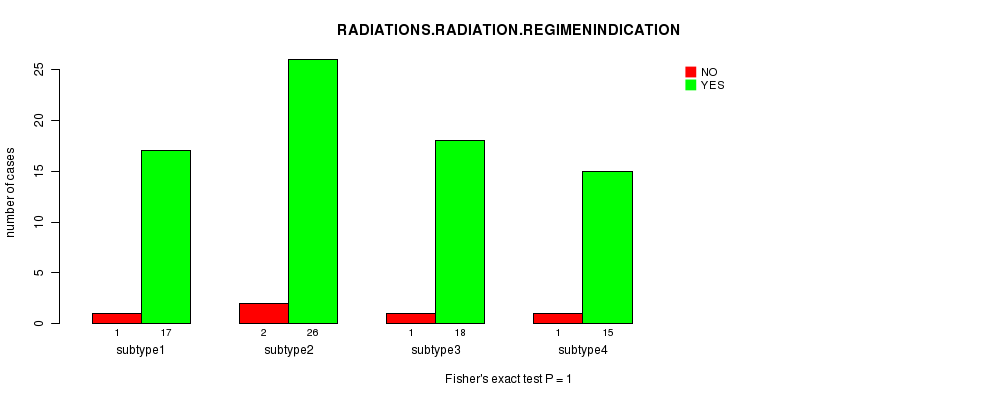
P value = 0.249 (Fisher's exact test)
Table S25. Clustering Approach #5: 'MIRseq CNMF subtypes' versus Clinical Feature #4: 'NEOADJUVANT.THERAPY'
nPatients | NO | YES |
---|---|---|
ALL | 3 | 78 |
subtype1 | 0 | 18 |
subtype2 | 3 | 25 |
subtype3 | 0 | 19 |
subtype4 | 0 | 16 |
Figure S20. Get High-res Image Clustering Approach #5: 'MIRseq CNMF subtypes' versus Clinical Feature #4: 'NEOADJUVANT.THERAPY'
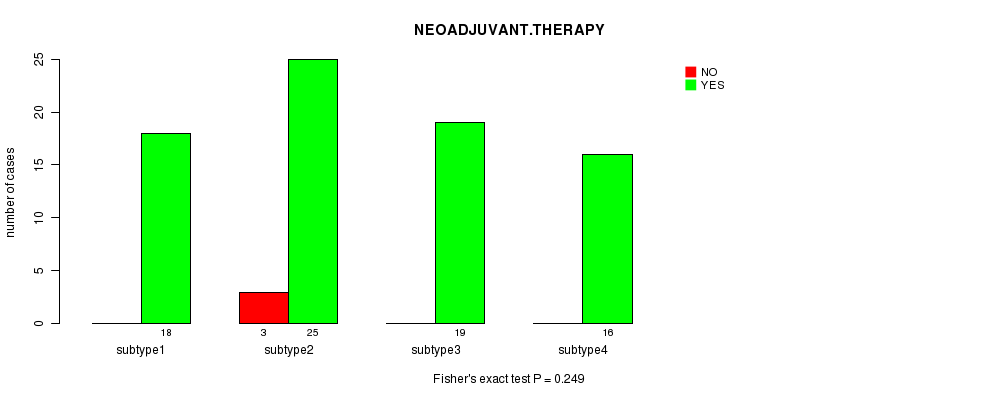
Table S26. Get Full Table Description of clustering approach #6: 'MIRseq cHierClus subtypes'
Cluster Labels | 1 | 2 | 3 |
---|---|---|---|
Number of samples | 15 | 32 | 34 |
P value = 1 (logrank test)
Table S27. Clustering Approach #6: 'MIRseq cHierClus subtypes' versus Clinical Feature #1: 'Time to Death'
nPatients | nDeath | Duration Range (Median), Month | |
---|---|---|---|
ALL | 81 | 0 | 0.9 - 65.9 (23.0) |
subtype1 | 15 | 0 | 3.0 - 47.4 (25.9) |
subtype2 | 32 | 0 | 0.9 - 65.9 (22.5) |
subtype3 | 34 | 0 | 1.0 - 54.9 (19.7) |
Figure S21. Get High-res Image Clustering Approach #6: 'MIRseq cHierClus subtypes' versus Clinical Feature #1: 'Time to Death'
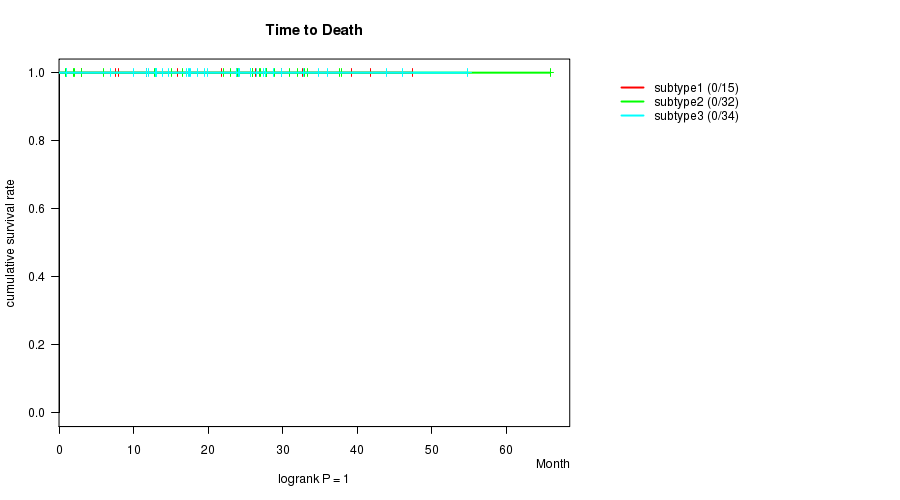
P value = 0.138 (ANOVA)
Table S28. Clustering Approach #6: 'MIRseq cHierClus subtypes' versus Clinical Feature #2: 'AGE'
nPatients | Mean (Std.Dev) | |
---|---|---|
ALL | 81 | 61.1 (6.7) |
subtype1 | 15 | 61.7 (6.1) |
subtype2 | 32 | 62.6 (6.9) |
subtype3 | 34 | 59.4 (6.6) |
Figure S22. Get High-res Image Clustering Approach #6: 'MIRseq cHierClus subtypes' versus Clinical Feature #2: 'AGE'
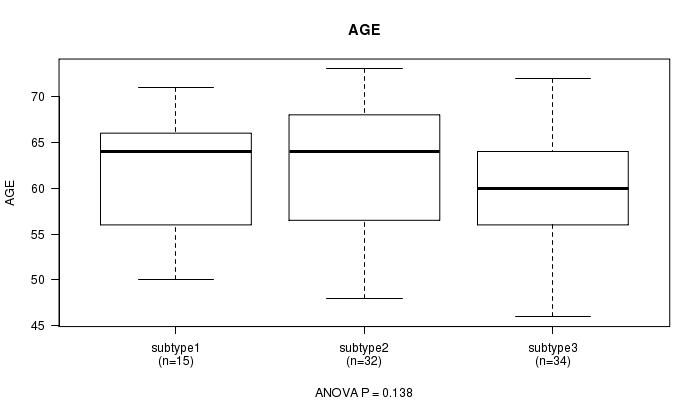
P value = 1 (Fisher's exact test)
Table S29. Clustering Approach #6: 'MIRseq cHierClus subtypes' versus Clinical Feature #3: 'RADIATIONS.RADIATION.REGIMENINDICATION'
nPatients | NO | YES |
---|---|---|
ALL | 5 | 76 |
subtype1 | 1 | 14 |
subtype2 | 2 | 30 |
subtype3 | 2 | 32 |
Figure S23. Get High-res Image Clustering Approach #6: 'MIRseq cHierClus subtypes' versus Clinical Feature #3: 'RADIATIONS.RADIATION.REGIMENINDICATION'
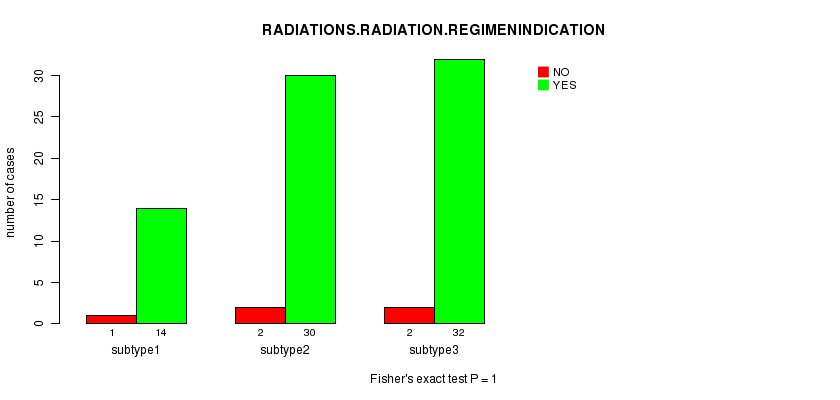
P value = 0.145 (Fisher's exact test)
Table S30. Clustering Approach #6: 'MIRseq cHierClus subtypes' versus Clinical Feature #4: 'NEOADJUVANT.THERAPY'
nPatients | NO | YES |
---|---|---|
ALL | 3 | 78 |
subtype1 | 0 | 15 |
subtype2 | 3 | 29 |
subtype3 | 0 | 34 |
Figure S24. Get High-res Image Clustering Approach #6: 'MIRseq cHierClus subtypes' versus Clinical Feature #4: 'NEOADJUVANT.THERAPY'
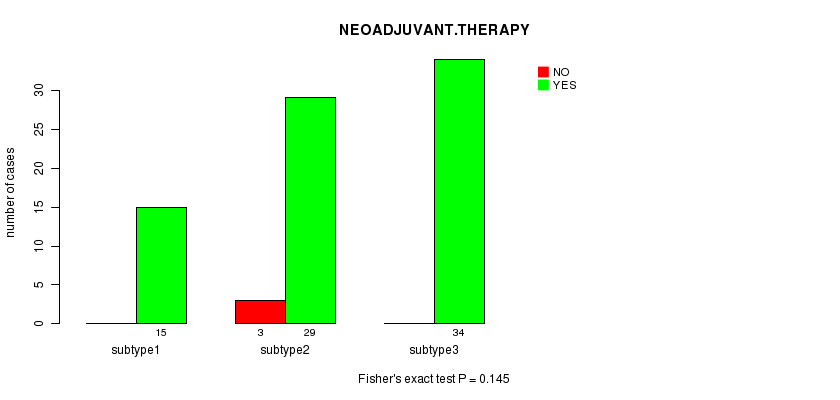
-
Cluster data file = PRAD.mergedcluster.txt
-
Clinical data file = PRAD.clin.merged.picked.txt
-
Number of patients = 124
-
Number of clustering approaches = 6
-
Number of selected clinical features = 4
-
Exclude small clusters that include fewer than K patients, K = 3
consensus non-negative matrix factorization clustering approach (Brunet et al. 2004)
Resampling-based clustering method (Monti et al. 2003)
For survival clinical features, the Kaplan-Meier survival curves of tumors with and without gene mutations were plotted and the statistical significance P values were estimated by logrank test (Bland and Altman 2004) using the 'survdiff' function in R
For continuous numerical clinical features, one-way analysis of variance (Howell 2002) was applied to compare the clinical values between tumor subtypes using 'anova' function in R
For binary clinical features, two-tailed Fisher's exact tests (Fisher 1922) were used to estimate the P values using the 'fisher.test' function in R
For multi-class clinical features (nominal or ordinal), Chi-square tests (Greenwood and Nikulin 1996) were used to estimate the P values using the 'chisq.test' function in R
This is an experimental feature. The full results of the analysis summarized in this report can be downloaded from the TCGA Data Coordination Center.