(primary solid tumor cohort)
This pipeline computes the correlation between cancer subtypes identified by different molecular patterns and selected clinical features.
Testing the association between subtypes identified by 6 different clustering approaches and 3 clinical features across 147 patients, one significant finding detected with P value < 0.05 and Q value < 0.25.
-
3 subtypes identified in current cancer cohort by 'CN CNMF'. These subtypes do not correlate to any clinical features.
-
3 subtypes identified in current cancer cohort by 'METHLYATION CNMF'. These subtypes correlate to 'AGE'.
-
CNMF clustering analysis on sequencing-based mRNA expression data identified 3 subtypes that do not correlate to any clinical features.
-
Consensus hierarchical clustering analysis on sequencing-based mRNA expression data identified 3 subtypes that do not correlate to any clinical features.
-
CNMF clustering analysis on sequencing-based miR expression data identified 4 subtypes that do not correlate to any clinical features.
-
Consensus hierarchical clustering analysis on sequencing-based miR expression data identified 3 subtypes that do not correlate to any clinical features.
Table 1. Get Full Table Overview of the association between subtypes identified by 6 different clustering approaches and 3 clinical features. Shown in the table are P values (Q values). Thresholded by P value < 0.05 and Q value < 0.25, one significant finding detected.
Clinical Features |
Time to Death |
AGE |
RADIATIONS RADIATION REGIMENINDICATION |
Statistical Tests | logrank test | ANOVA | Fisher's exact test |
CN CNMF |
100 (1.00) |
0.0257 (0.436) |
0.853 (1.00) |
METHLYATION CNMF |
100 (1.00) |
0.00439 (0.0789) |
0.861 (1.00) |
RNAseq CNMF subtypes |
100 (1.00) |
0.207 (1.00) |
0.86 (1.00) |
RNAseq cHierClus subtypes |
100 (1.00) |
0.302 (1.00) |
1 (1.00) |
MIRseq CNMF subtypes |
100 (1.00) |
0.0775 (1.00) |
0.631 (1.00) |
MIRseq cHierClus subtypes |
100 (1.00) |
0.46 (1.00) |
0.197 (1.00) |
Table S1. Get Full Table Description of clustering approach #1: 'CN CNMF'
Cluster Labels | 1 | 2 | 3 | 4 |
---|---|---|---|---|
Number of samples | 33 | 66 | 46 | 1 |
P value = 100 (logrank test), Q value = 1
Table S2. Clustering Approach #1: 'CN CNMF' versus Clinical Feature #1: 'Time to Death'
nPatients | nDeath | Duration Range (Median), Month | |
---|---|---|---|
ALL | 145 | 1 | 0.3 - 66.0 (16.8) |
subtype1 | 33 | 0 | 0.3 - 63.3 (9.4) |
subtype2 | 66 | 0 | 1.0 - 65.9 (17.6) |
subtype3 | 46 | 1 | 0.9 - 66.0 (18.5) |
Figure S1. Get High-res Image Clustering Approach #1: 'CN CNMF' versus Clinical Feature #1: 'Time to Death'
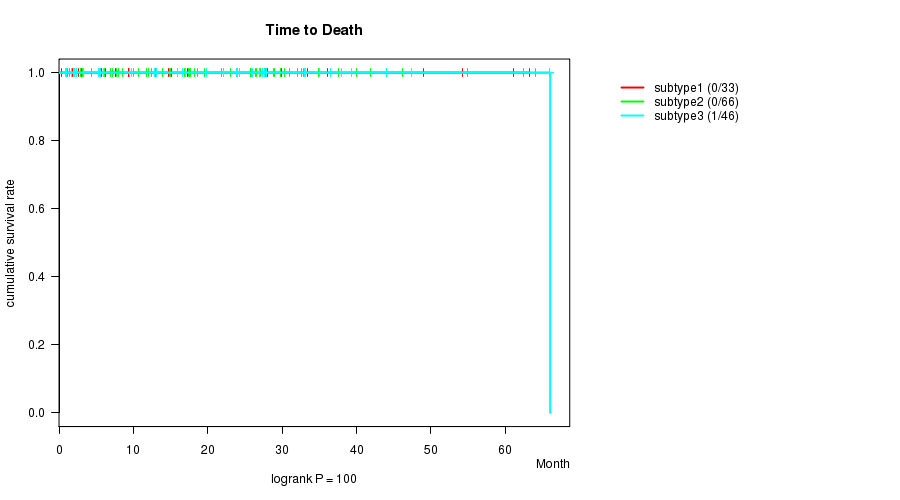
P value = 0.0257 (ANOVA), Q value = 0.44
Table S3. Clustering Approach #1: 'CN CNMF' versus Clinical Feature #2: 'AGE'
nPatients | Mean (Std.Dev) | |
---|---|---|
ALL | 145 | 60.5 (6.9) |
subtype1 | 33 | 61.9 (6.1) |
subtype2 | 66 | 58.8 (7.5) |
subtype3 | 46 | 61.9 (6.1) |
Figure S2. Get High-res Image Clustering Approach #1: 'CN CNMF' versus Clinical Feature #2: 'AGE'
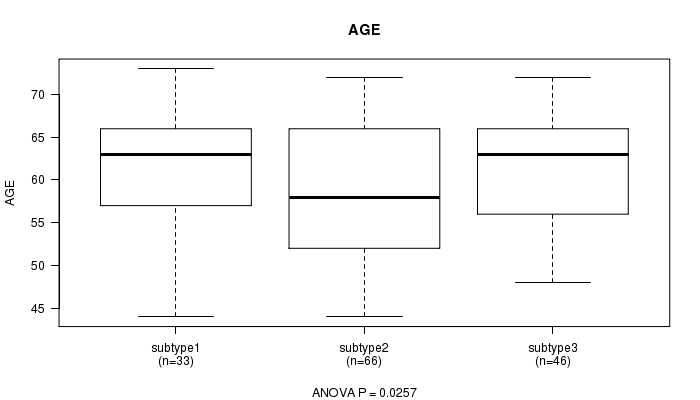
P value = 0.853 (Fisher's exact test), Q value = 1
Table S4. Clustering Approach #1: 'CN CNMF' versus Clinical Feature #3: 'RADIATIONS.RADIATION.REGIMENINDICATION'
nPatients | NO | YES |
---|---|---|
ALL | 5 | 140 |
subtype1 | 1 | 32 |
subtype2 | 3 | 63 |
subtype3 | 1 | 45 |
Figure S3. Get High-res Image Clustering Approach #1: 'CN CNMF' versus Clinical Feature #3: 'RADIATIONS.RADIATION.REGIMENINDICATION'
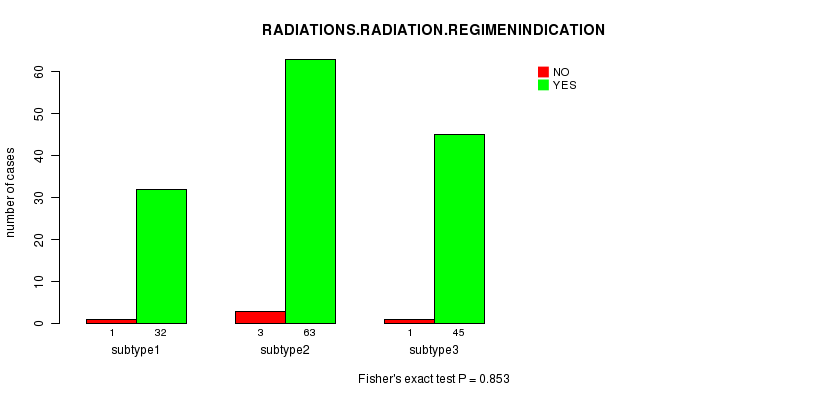
Table S5. Get Full Table Description of clustering approach #2: 'METHLYATION CNMF'
Cluster Labels | 1 | 2 | 3 |
---|---|---|---|
Number of samples | 47 | 43 | 57 |
P value = 100 (logrank test), Q value = 1
Table S6. Clustering Approach #2: 'METHLYATION CNMF' versus Clinical Feature #1: 'Time to Death'
nPatients | nDeath | Duration Range (Median), Month | |
---|---|---|---|
ALL | 147 | 1 | 0.3 - 66.0 (16.6) |
subtype1 | 47 | 0 | 0.3 - 65.9 (15.9) |
subtype2 | 43 | 0 | 1.1 - 54.9 (13.1) |
subtype3 | 57 | 1 | 1.0 - 66.0 (17.5) |
Figure S4. Get High-res Image Clustering Approach #2: 'METHLYATION CNMF' versus Clinical Feature #1: 'Time to Death'
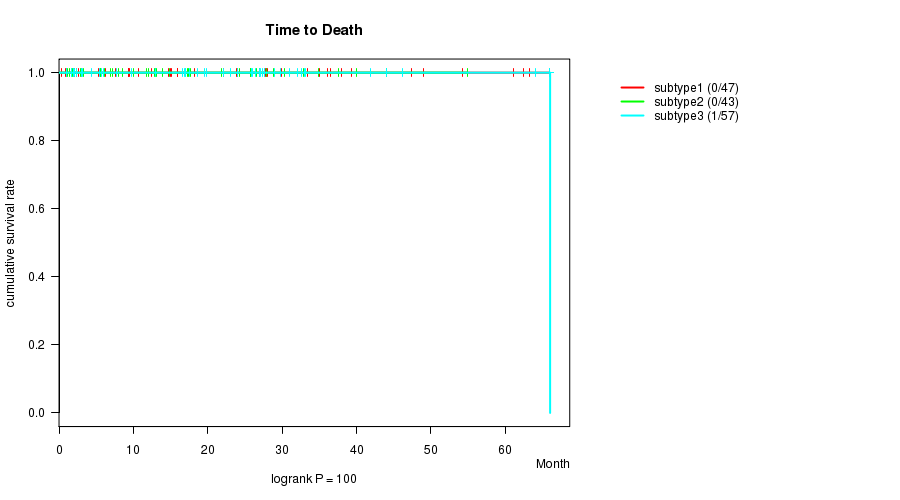
P value = 0.00439 (ANOVA), Q value = 0.079
Table S7. Clustering Approach #2: 'METHLYATION CNMF' versus Clinical Feature #2: 'AGE'
nPatients | Mean (Std.Dev) | |
---|---|---|
ALL | 147 | 60.4 (7.0) |
subtype1 | 47 | 61.9 (6.3) |
subtype2 | 43 | 57.5 (7.2) |
subtype3 | 57 | 61.4 (6.8) |
Figure S5. Get High-res Image Clustering Approach #2: 'METHLYATION CNMF' versus Clinical Feature #2: 'AGE'
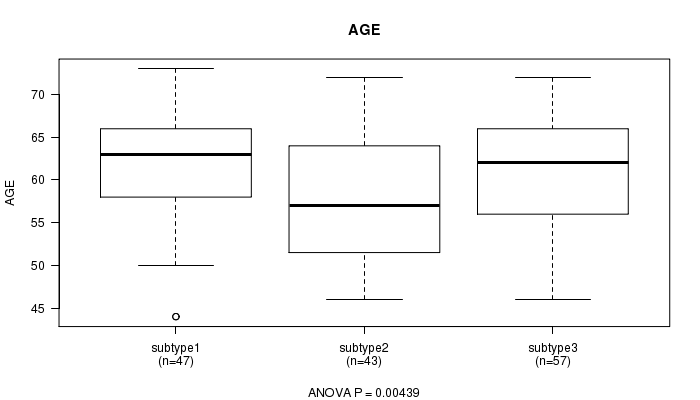
P value = 0.861 (Fisher's exact test), Q value = 1
Table S8. Clustering Approach #2: 'METHLYATION CNMF' versus Clinical Feature #3: 'RADIATIONS.RADIATION.REGIMENINDICATION'
nPatients | NO | YES |
---|---|---|
ALL | 5 | 142 |
subtype1 | 1 | 46 |
subtype2 | 2 | 41 |
subtype3 | 2 | 55 |
Figure S6. Get High-res Image Clustering Approach #2: 'METHLYATION CNMF' versus Clinical Feature #3: 'RADIATIONS.RADIATION.REGIMENINDICATION'
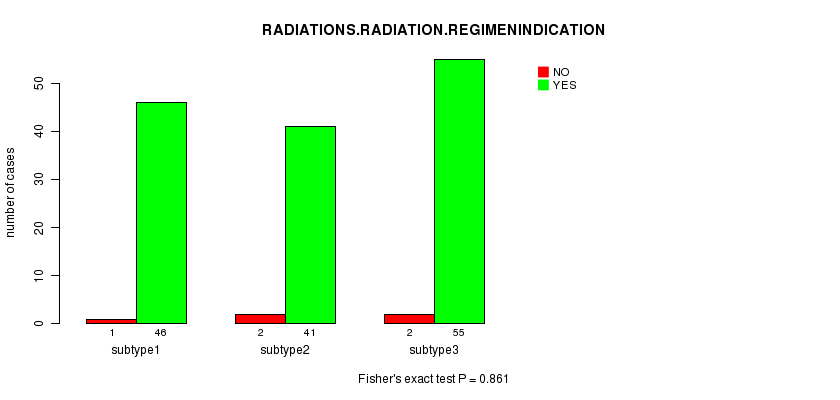
Table S9. Get Full Table Description of clustering approach #3: 'RNAseq CNMF subtypes'
Cluster Labels | 1 | 2 | 3 |
---|---|---|---|
Number of samples | 40 | 35 | 42 |
P value = 100 (logrank test), Q value = 1
Table S10. Clustering Approach #3: 'RNAseq CNMF subtypes' versus Clinical Feature #1: 'Time to Death'
nPatients | nDeath | Duration Range (Median), Month | |
---|---|---|---|
ALL | 117 | 1 | 0.3 - 66.0 (14.8) |
subtype1 | 40 | 0 | 0.3 - 65.9 (16.0) |
subtype2 | 35 | 0 | 1.0 - 54.9 (13.0) |
subtype3 | 42 | 1 | 1.0 - 66.0 (14.8) |
Figure S7. Get High-res Image Clustering Approach #3: 'RNAseq CNMF subtypes' versus Clinical Feature #1: 'Time to Death'
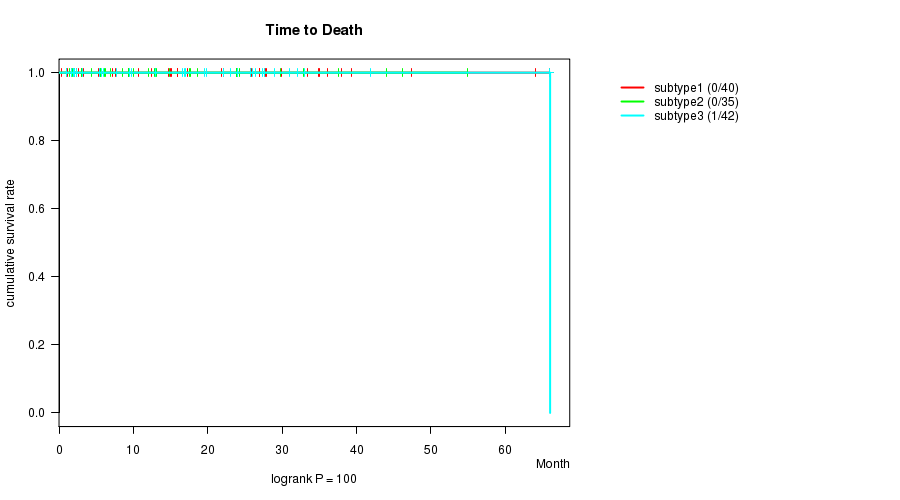
P value = 0.207 (ANOVA), Q value = 1
Table S11. Clustering Approach #3: 'RNAseq CNMF subtypes' versus Clinical Feature #2: 'AGE'
nPatients | Mean (Std.Dev) | |
---|---|---|
ALL | 117 | 60.7 (7.2) |
subtype1 | 40 | 61.5 (6.9) |
subtype2 | 35 | 58.9 (7.3) |
subtype3 | 42 | 61.4 (7.2) |
Figure S8. Get High-res Image Clustering Approach #3: 'RNAseq CNMF subtypes' versus Clinical Feature #2: 'AGE'
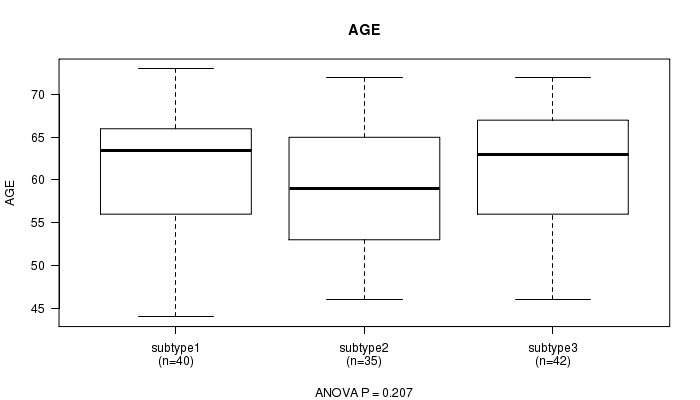
P value = 0.86 (Fisher's exact test), Q value = 1
Table S12. Clustering Approach #3: 'RNAseq CNMF subtypes' versus Clinical Feature #3: 'RADIATIONS.RADIATION.REGIMENINDICATION'
nPatients | NO | YES |
---|---|---|
ALL | 5 | 112 |
subtype1 | 1 | 39 |
subtype2 | 2 | 33 |
subtype3 | 2 | 40 |
Figure S9. Get High-res Image Clustering Approach #3: 'RNAseq CNMF subtypes' versus Clinical Feature #3: 'RADIATIONS.RADIATION.REGIMENINDICATION'
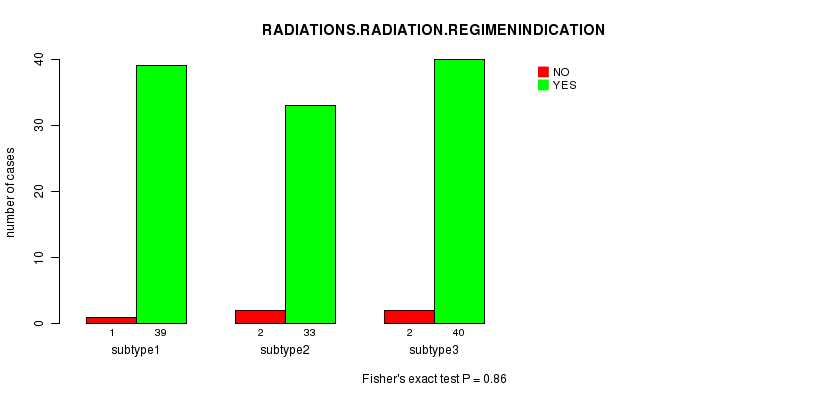
Table S13. Get Full Table Description of clustering approach #4: 'RNAseq cHierClus subtypes'
Cluster Labels | 1 | 2 | 3 |
---|---|---|---|
Number of samples | 34 | 36 | 47 |
P value = 100 (logrank test), Q value = 1
Table S14. Clustering Approach #4: 'RNAseq cHierClus subtypes' versus Clinical Feature #1: 'Time to Death'
nPatients | nDeath | Duration Range (Median), Month | |
---|---|---|---|
ALL | 117 | 1 | 0.3 - 66.0 (14.8) |
subtype1 | 34 | 0 | 1.0 - 65.9 (15.5) |
subtype2 | 36 | 1 | 1.0 - 66.0 (13.0) |
subtype3 | 47 | 0 | 0.3 - 54.9 (13.1) |
Figure S10. Get High-res Image Clustering Approach #4: 'RNAseq cHierClus subtypes' versus Clinical Feature #1: 'Time to Death'
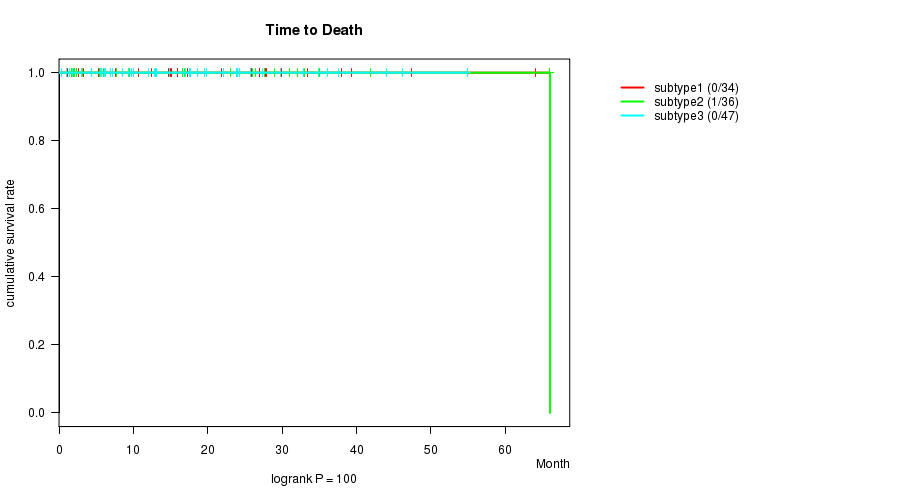
P value = 0.302 (ANOVA), Q value = 1
Table S15. Clustering Approach #4: 'RNAseq cHierClus subtypes' versus Clinical Feature #2: 'AGE'
nPatients | Mean (Std.Dev) | |
---|---|---|
ALL | 117 | 60.7 (7.2) |
subtype1 | 34 | 60.9 (7.1) |
subtype2 | 36 | 62.0 (7.5) |
subtype3 | 47 | 59.5 (6.9) |
Figure S11. Get High-res Image Clustering Approach #4: 'RNAseq cHierClus subtypes' versus Clinical Feature #2: 'AGE'
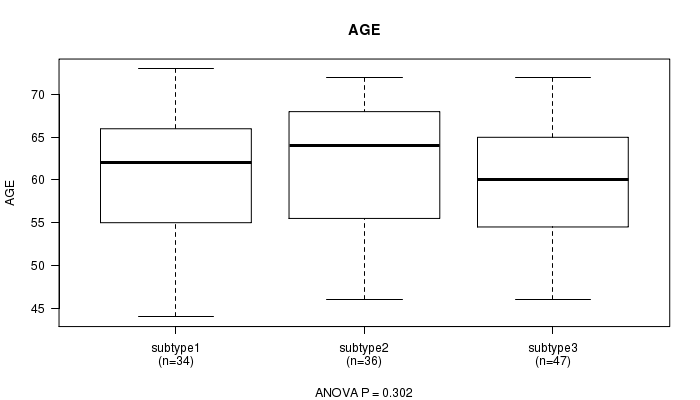
P value = 1 (Fisher's exact test), Q value = 1
Table S16. Clustering Approach #4: 'RNAseq cHierClus subtypes' versus Clinical Feature #3: 'RADIATIONS.RADIATION.REGIMENINDICATION'
nPatients | NO | YES |
---|---|---|
ALL | 5 | 112 |
subtype1 | 1 | 33 |
subtype2 | 2 | 34 |
subtype3 | 2 | 45 |
Figure S12. Get High-res Image Clustering Approach #4: 'RNAseq cHierClus subtypes' versus Clinical Feature #3: 'RADIATIONS.RADIATION.REGIMENINDICATION'
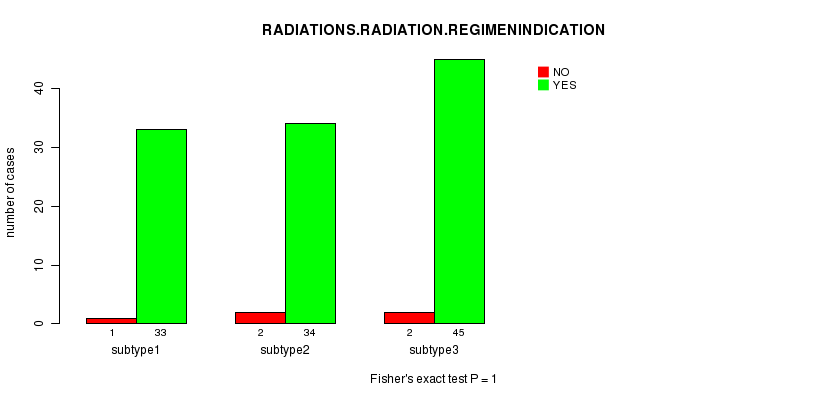
Table S17. Get Full Table Description of clustering approach #5: 'MIRseq CNMF subtypes'
Cluster Labels | 1 | 2 | 3 | 4 |
---|---|---|---|---|
Number of samples | 41 | 40 | 26 | 38 |
P value = 100 (logrank test), Q value = 1
Table S18. Clustering Approach #5: 'MIRseq CNMF subtypes' versus Clinical Feature #1: 'Time to Death'
nPatients | nDeath | Duration Range (Median), Month | |
---|---|---|---|
ALL | 145 | 1 | 0.3 - 66.0 (16.6) |
subtype1 | 41 | 0 | 0.9 - 66.0 (22.1) |
subtype2 | 40 | 0 | 3.0 - 54.9 (19.7) |
subtype3 | 26 | 0 | 0.3 - 64.1 (16.0) |
subtype4 | 38 | 1 | 1.0 - 66.0 (5.7) |
Figure S13. Get High-res Image Clustering Approach #5: 'MIRseq CNMF subtypes' versus Clinical Feature #1: 'Time to Death'
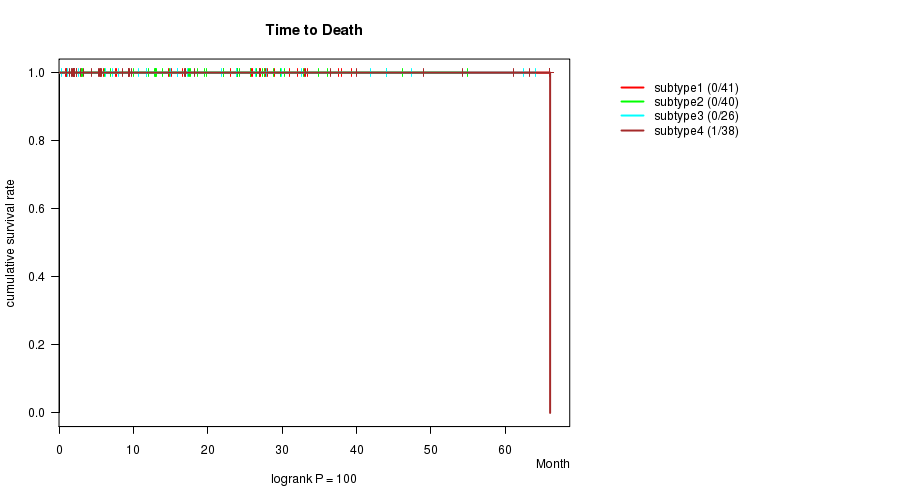
P value = 0.0775 (ANOVA), Q value = 1
Table S19. Clustering Approach #5: 'MIRseq CNMF subtypes' versus Clinical Feature #2: 'AGE'
nPatients | Mean (Std.Dev) | |
---|---|---|
ALL | 145 | 60.5 (6.9) |
subtype1 | 41 | 62.7 (6.1) |
subtype2 | 40 | 58.9 (7.0) |
subtype3 | 26 | 59.4 (6.8) |
subtype4 | 38 | 60.4 (7.4) |
Figure S14. Get High-res Image Clustering Approach #5: 'MIRseq CNMF subtypes' versus Clinical Feature #2: 'AGE'
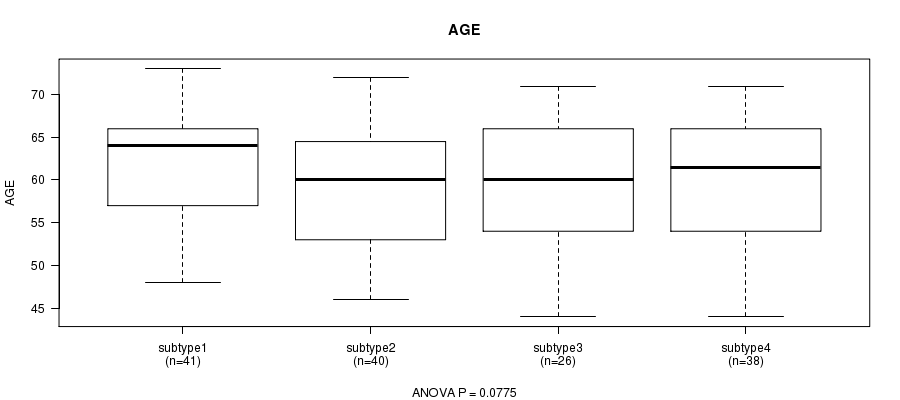
P value = 0.631 (Fisher's exact test), Q value = 1
Table S20. Clustering Approach #5: 'MIRseq CNMF subtypes' versus Clinical Feature #3: 'RADIATIONS.RADIATION.REGIMENINDICATION'
nPatients | NO | YES |
---|---|---|
ALL | 5 | 140 |
subtype1 | 2 | 39 |
subtype2 | 2 | 38 |
subtype3 | 1 | 25 |
subtype4 | 0 | 38 |
Figure S15. Get High-res Image Clustering Approach #5: 'MIRseq CNMF subtypes' versus Clinical Feature #3: 'RADIATIONS.RADIATION.REGIMENINDICATION'
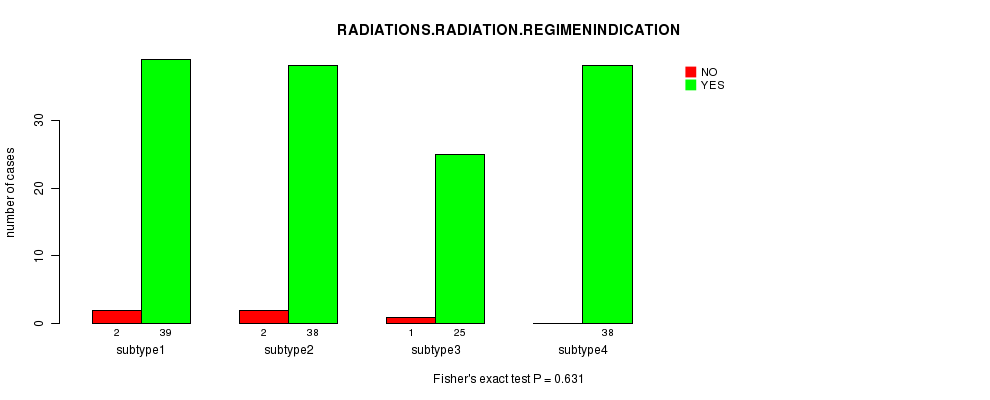
Table S21. Get Full Table Description of clustering approach #6: 'MIRseq cHierClus subtypes'
Cluster Labels | 1 | 2 | 3 |
---|---|---|---|
Number of samples | 25 | 68 | 52 |
P value = 100 (logrank test), Q value = 1
Table S22. Clustering Approach #6: 'MIRseq cHierClus subtypes' versus Clinical Feature #1: 'Time to Death'
nPatients | nDeath | Duration Range (Median), Month | |
---|---|---|---|
ALL | 145 | 1 | 0.3 - 66.0 (16.6) |
subtype1 | 25 | 0 | 0.3 - 64.1 (18.2) |
subtype2 | 68 | 0 | 0.9 - 65.9 (22.6) |
subtype3 | 52 | 1 | 1.0 - 66.0 (6.0) |
Figure S16. Get High-res Image Clustering Approach #6: 'MIRseq cHierClus subtypes' versus Clinical Feature #1: 'Time to Death'
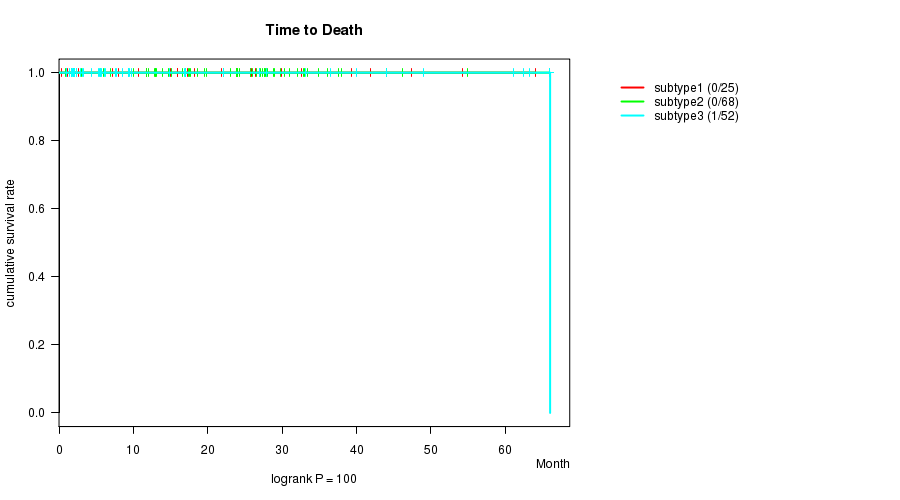
P value = 0.46 (ANOVA), Q value = 1
Table S23. Clustering Approach #6: 'MIRseq cHierClus subtypes' versus Clinical Feature #2: 'AGE'
nPatients | Mean (Std.Dev) | |
---|---|---|
ALL | 145 | 60.5 (6.9) |
subtype1 | 25 | 59.0 (6.7) |
subtype2 | 68 | 61.0 (6.9) |
subtype3 | 52 | 60.4 (7.2) |
Figure S17. Get High-res Image Clustering Approach #6: 'MIRseq cHierClus subtypes' versus Clinical Feature #2: 'AGE'
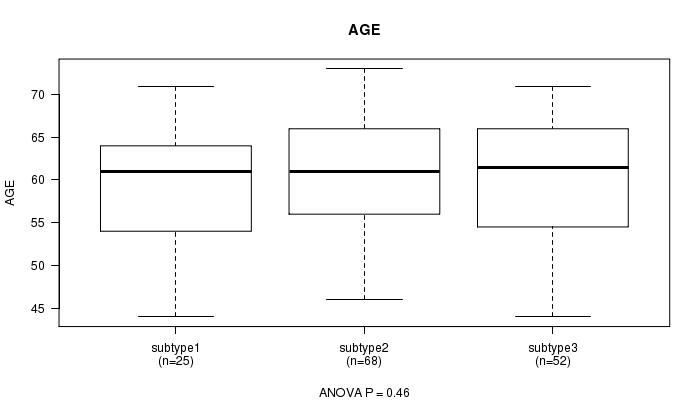
P value = 0.197 (Fisher's exact test), Q value = 1
Table S24. Clustering Approach #6: 'MIRseq cHierClus subtypes' versus Clinical Feature #3: 'RADIATIONS.RADIATION.REGIMENINDICATION'
nPatients | NO | YES |
---|---|---|
ALL | 5 | 140 |
subtype1 | 1 | 24 |
subtype2 | 4 | 64 |
subtype3 | 0 | 52 |
Figure S18. Get High-res Image Clustering Approach #6: 'MIRseq cHierClus subtypes' versus Clinical Feature #3: 'RADIATIONS.RADIATION.REGIMENINDICATION'
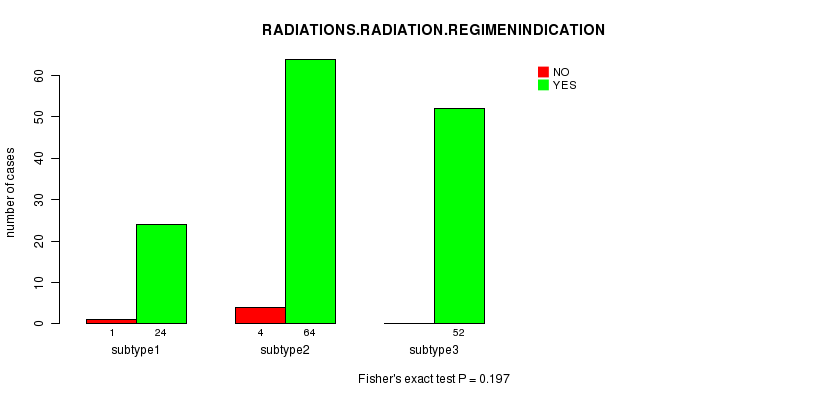
-
Cluster data file = PRAD-TP.mergedcluster.txt
-
Clinical data file = PRAD-TP.clin.merged.picked.txt
-
Number of patients = 147
-
Number of clustering approaches = 6
-
Number of selected clinical features = 3
-
Exclude small clusters that include fewer than K patients, K = 3
consensus non-negative matrix factorization clustering approach (Brunet et al. 2004)
Resampling-based clustering method (Monti et al. 2003)
For survival clinical features, the Kaplan-Meier survival curves of tumors with and without gene mutations were plotted and the statistical significance P values were estimated by logrank test (Bland and Altman 2004) using the 'survdiff' function in R
For continuous numerical clinical features, one-way analysis of variance (Howell 2002) was applied to compare the clinical values between tumor subtypes using 'anova' function in R
For binary clinical features, two-tailed Fisher's exact tests (Fisher 1922) were used to estimate the P values using the 'fisher.test' function in R
For multiple hypothesis correction, Q value is the False Discovery Rate (FDR) analogue of the P value (Benjamini and Hochberg 1995), defined as the minimum FDR at which the test may be called significant. We used the 'Benjamini and Hochberg' method of 'p.adjust' function in R to convert P values into Q values.
This is an experimental feature. The full results of the analysis summarized in this report can be downloaded from the TCGA Data Coordination Center.