This pipeline uses various statistical tests to identify genes whose promoter methylation levels correlated to selected clinical features.
Testing the association between 13024 genes and 4 clinical features across 261 samples, statistically thresholded by Q value < 0.05, 1 clinical feature related to at least one genes.
-
13 genes correlated to 'AGE'.
-
C16ORF45 , PCDHGA3 , PCDHGA8 , PCDHGB3 , PCDHGB6 , ...
-
No genes correlated to 'Time to Death', 'KARNOFSKY.PERFORMANCE.SCORE', and 'TUMOR.STAGE'.
Complete statistical result table is provided in Supplement Table 1
Table 1. Get Full Table This table shows the clinical features, statistical methods used, and the number of genes that are significantly associated with each clinical feature at Q value < 0.05.
Clinical feature | Statistical test | Significant genes | Associated with | Associated with | ||
---|---|---|---|---|---|---|
Time to Death | Cox regression test | N=0 | ||||
AGE | Spearman correlation test | N=13 | older | N=5 | younger | N=8 |
KARNOFSKY PERFORMANCE SCORE | Spearman correlation test | N=0 | ||||
TUMOR STAGE | Spearman correlation test | N=0 |
Table S1. Basic characteristics of clinical feature: 'Time to Death'
Time to Death | Duration (Months) | 0.3-180.2 (median=28.2) |
censored | N = 112 | |
death | N = 147 | |
Significant markers | N = 0 |
Table S2. Basic characteristics of clinical feature: 'AGE'
AGE | Mean (SD) | 58.95 (11) |
Significant markers | N = 13 | |
pos. correlated | 5 | |
neg. correlated | 8 |
Table S3. Get Full Table List of top 10 genes significantly correlated to 'AGE' by Spearman correlation test
SpearmanCorr | corrP | Q | |
---|---|---|---|
C16ORF45 | -0.3202 | 1.835e-07 | 0.00239 |
PCDHGA3 | 0.3176 | 2.317e-07 | 0.00302 |
PCDHGA8 | 0.3176 | 2.317e-07 | 0.00302 |
PCDHGB3 | 0.3176 | 2.317e-07 | 0.00302 |
PCDHGB6 | 0.3143 | 3.145e-07 | 0.00409 |
PCDHGB7 | 0.3143 | 3.145e-07 | 0.00409 |
KLK12 | -0.3141 | 3.194e-07 | 0.00416 |
PDZK1 | -0.3095 | 5.679e-07 | 0.00739 |
KCNIP1 | -0.3031 | 8.536e-07 | 0.0111 |
VWA5B1 | -0.2914 | 2.302e-06 | 0.03 |
Figure S1. Get High-res Image As an example, this figure shows the association of C16ORF45 to 'AGE'. P value = 1.83e-07 with Spearman correlation analysis. The straight line presents the best linear regression.
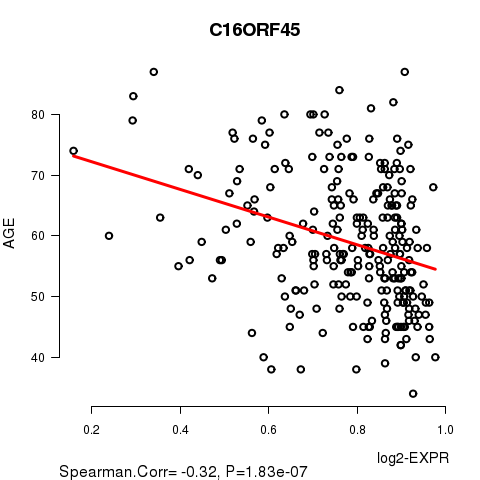
No gene related to 'KARNOFSKY.PERFORMANCE.SCORE'.
Table S4. Basic characteristics of clinical feature: 'KARNOFSKY.PERFORMANCE.SCORE'
KARNOFSKY.PERFORMANCE.SCORE | Mean (SD) | 74.29 (12) |
Score | N | |
60 | 5 | |
80 | 8 | |
100 | 1 | |
Significant markers | N = 0 |
-
Expresson data file = OV-TP.meth.for_correlation.filtered_data.txt
-
Clinical data file = OV-TP.clin.merged.picked.txt
-
Number of patients = 261
-
Number of genes = 13024
-
Number of clinical features = 4
For survival clinical features, Wald's test in univariate Cox regression analysis with proportional hazards model (Andersen and Gill 1982) was used to estimate the P values using the 'coxph' function in R. Kaplan-Meier survival curves were plot using the four quartile subgroups of patients based on expression levels
For continuous numerical clinical features, Spearman's rank correlation coefficients (Spearman 1904) and two-tailed P values were estimated using 'cor.test' function in R
For multiple hypothesis correction, Q value is the False Discovery Rate (FDR) analogue of the P value (Benjamini and Hochberg 1995), defined as the minimum FDR at which the test may be called significant. We used the 'Benjamini and Hochberg' method of 'p.adjust' function in R to convert P values into Q values.
This is an experimental feature. The full results of the analysis summarized in this report can be downloaded from the TCGA Data Coordination Center.